eBird
eBird began with a simple idea—that every birdwatcher has unique knowledge and experience. Our goal is to gather this information in the form of checklists of birds, archive it, and freely share it to power new data-driven approaches to science, conservation and education. At the same time, we develop tools that make birding more rewarding. From being able to manage lists, photos and audio recordings, to seeing real-time maps of species distribution, to alerts that let you know when species have been seen, we strive to provide the most current and useful information to the birding community.
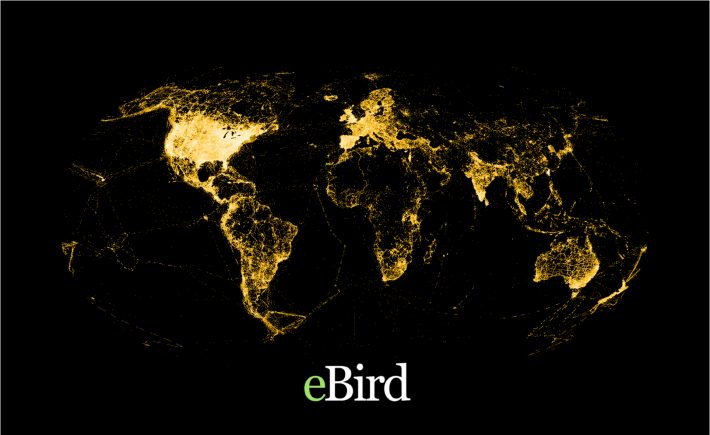
eBird is among the world’s largest biodiversity-related science projects, with more than 100 million bird sightings contributed annually by eBirders around the world and an average participation growth rate of approximately 20% year over year. A collaborative enterprise with hundreds of partner organizations, thousands of regional experts, and hundreds of thousands of users, eBird is managed by the Cornell Lab of Ornithology.
eBird data document bird distribution, abundance, habitat use, and trends through checklist data collected within a simple, scientific framework. Birders enter when, where, and how they went birding, and then fill out a checklist of all the birds seen and heard during the outing. eBird’s free mobile app allows offline data collection anywhere in the world, and the website provides many ways to explore and summarize your data and other observations from the global eBird community.
eBird is available worldwide, comprising a network of local, national, and international partners. eBird directly collaborates with hundreds of partner groups for regional data entry portals, outreach, engagement, and local impact.
Links
To discover more about eBird, their work and how to get involved check out their website or the introductory video below.
Selected Publications
La Sorte, F. A. and C. H. Graham (2020). “Phenological synchronization of seasonal bird migration with vegetation greenness across dietary guilds”. Journal of Animal Ecology.
Callaghan, C. T., R. E. Major, M. B. Lyons, J. M. Martin, J. H. Wilshire, R. T. Kingsford and W. K. Cornwell (2019). “Using citizen science data to define and track restoration targets in urban areas.” Journal of Applied Ecology.
Miller, D. A. W., K. Pacifici, J. S. Sanderlin, B. J. Reich and B. Gardner (2019). “The recent past and promising future for data integration methods to estimate species’ distributions.” Methods in Ecology and Evolution 10(1): 22-37.
Integrating auxiliary data in optimal spatial design for species distribution modeling. Methods Ecol Evol. 2018; 9: 1626– 1637.
, , .Fournier, A. M. V., A. R. Sullivan, J. K. Bump, M. Perkins, M. C. Shieldcastle, S. L. King and D. Thompson (2017). “Combining citizen science species distribution models and stable isotopes reveals migratory connectivity in the secretive Virginia rail.” Journal of Applied Ecology 54(2): 618-627.
Estimates of observer expertise improve species distributions from citizen science data. Methods Ecol Evol. 2018; 9: 88– 97.
, , , .Schmitt, S., R. Pouteau, D. Justeau, F. de Boissieu, P. Birnbaum and N. Golding (2017). “ssdm: An r package to predict distribution of species richness and composition based on stacked species distribution models.” Methods in Ecology and Evolution 8(12): 1795-1803.
Munson, M.A., Caruana, R., Fink, D., Hochachka, W.M., Iliff, M., Rosenberg, K.V., Sheldon, D., Sullivan, B.L., Wood, C. and Kelling, S. (2010), A method for measuring the relative information content of data from different monitoring protocols. Methods in Ecology and Evolution, 1: 263-273.
Full list of publications here.
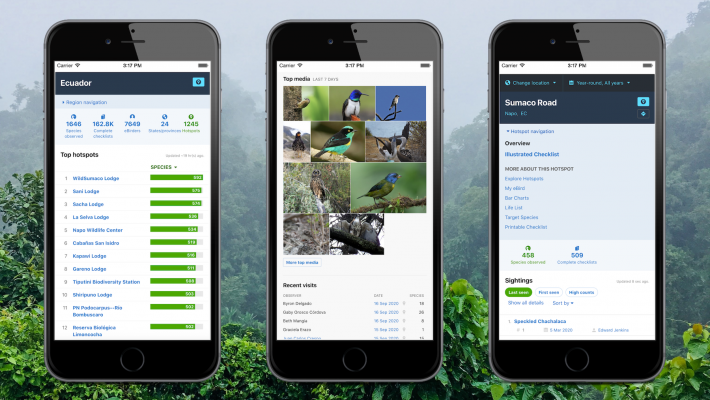
Like what we stand for?
Support our mission and help develop the next generation of ecologists by donating to the British Ecological Society.